Health and activity monitoring by wearables in extreme conditions
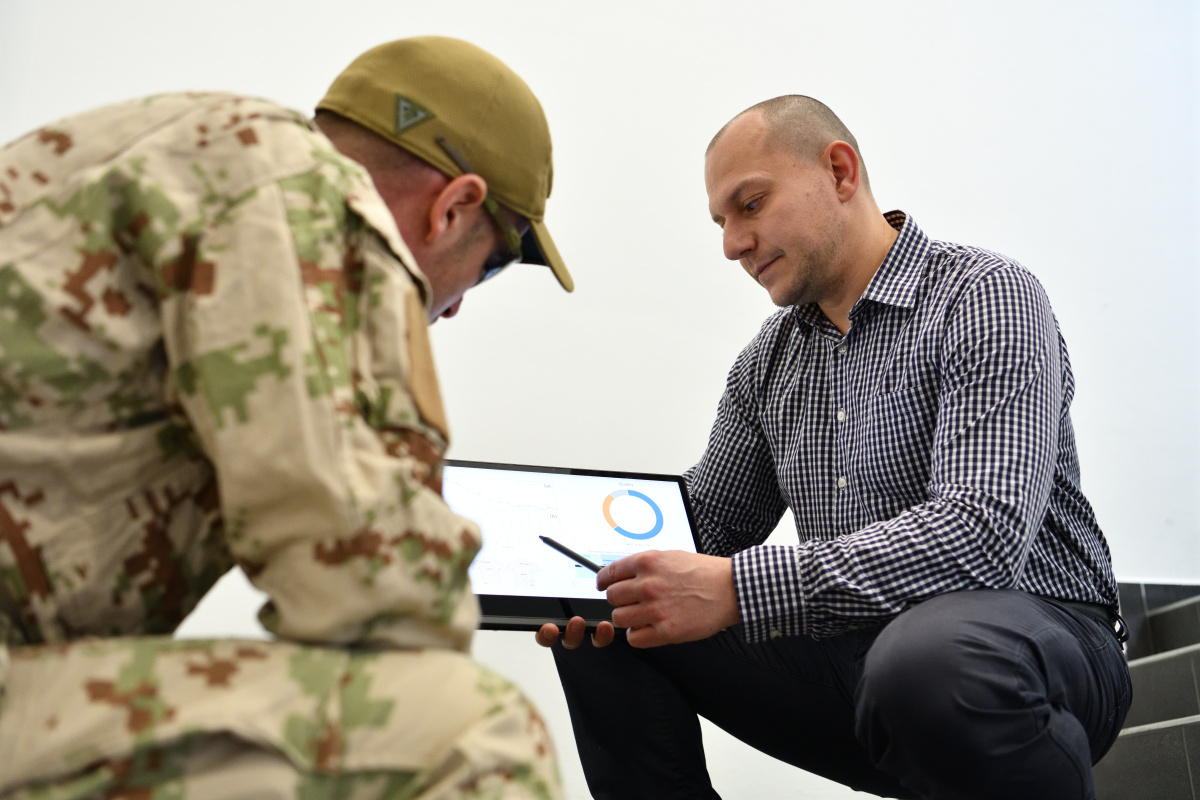
The project focused on advancing the analysis of biosignals from wearables to enable real-time monitoring in free-living and extreme conditions, such as those experienced by firefighters or soldiers. The primary objective was to develop computationally efficient methods for classifying biosignals, such as ECG, PPG, and motion data, based on their quality and usability for subsequent analysis. By tailoring analysis techniques to the assessed signal quality, it aimed to enhance diagnostic accuracy and maximize the usability of data collected under challenging conditions. This research directly addressed the growing demand for reliable self-monitoring tools in wearable technology, particularly for cardiovascular health.
The research led to impactful results, including a robust algorithm for real-time ECG signal quality assessment (Smital et al., IEEE TBME, 2020, DOI: 10.1109/TBME.2020.2969719), specifically designed for long-term recordings. A complementary resource was also developed: a comprehensive database of long-term ECG signals annotated with detailed quality metrics, intended as a reference for developing similar algorithms (Nemcova et al., PhysioNet, 2020, DOI: 10.13026/kah4-0w24). Significant progress was achieved in detecting P-waves in ECG signals with diverse pathologies and arrhythmias leading to several high-impact publications (Marsanova et al., Scientific Reports, 2019, DOI: 10.1038/s41598-019-55323-3, Saclova et al., Scientific Reports, 2022, DOI: 10.1038/s41598-022-10656-4, Saclova et al., Physiological Measurement, 2022, DOI: 10.1088/1361-6579/ac944e). Additionally, the team provided precise P-wave annotations for a widely used standard database of ECG signals, further supporting the research community (Marsanova et al., PhysioNet, 2021, DOI: 10.13026/hwvj-5b53).
The project's scope extended to PPG analysis, particularly exploring the feasibility of monitoring heart rate, blood oxygen saturation, and blood pressure via smartphones (Nemcova et al., Biomedical Signal Processing and Control, 2020, DOI: 10.1016/j.bspc.2020.101928). The results highlighted the potential for real-time monitoring in mobile health applications. This effort was complemented by a database of smartphone-acquired PPG signals annotated for quality (Nemcova et al., PhysioNet, 2021, DOI: 10.13026/tn53-8153, Nemcova et al., BioMed Research International, 2021, DOI: 10.1155/2021/3453007), enabling further algorithmic development within the research community.
These results provide a solid foundation for integrating multi-signal approaches into real-world applications, particularly in dynamic and demanding environments. Collaboration with the Mayo Clinic was instrumental in ensuring the clinical relevance of the findings and their translation into practical health monitoring solutions.
This project laid a strong foundation for biosignal analysis in wearable technologies, and the research continues within the follow-up project Advanced Signal Processing Framework for Sensor Networks (ONR, #N00014-23-S-B001).
ONR USA